
Causal AI in business risk management – the Insurance paradigm
15 Απριλίου 2025

Causal AI in business risk management – the Insurance paradigm

Causal AI in business risk management – the Insurance paradigm
Causal AI in business risk management – the Insurance paradigm
Causal AI in business risk management – the Insurance paradigm
Causal AI in business risk management – the Insurance paradigm
Konstantia Moirogiorgou, Head of R&D, Seven Red Lines
With Artificial Intelligence (AI) transforming almost every domain of applications related to automated decision-making processes, it is crucial to design models that are transparent and interpretable. Fields like Causal Machine Learning (Causal ML) and Explainable AI (XAI) provide ways to improve the performance of AI models beyond finding patterns and correlations in data, by understanding how AI reasons, learns and makes decisions.
XAI employs post-hoc explainability techniques generating model-agnostic explanations for feature importance and interaction effects. The disadvantage here is that post-hoc explainability provides insights into the decision-making process after the trained ML model has made predictions.
Causal ML, on the other hand, focuses on understanding cause-and-effect relationships generating explanations earlier in the AI pipeline, allowing them to impose rules and restrictions during the model building.
With Artificial Intelligence (AI) transforming almost every domain of applications related to automated decision-making processes, it is crucial to design models that are transparent and interpretable. Fields like Causal Machine Learning (Causal ML) and Explainable AI (XAI) provide ways to improve the performance of AI models beyond finding patterns and correlations in data, by understanding how AI reasons, learns and makes decisions.
XAI employs post-hoc explainability techniques generating model-agnostic explanations for feature importance and interaction effects. The disadvantage here is that post-hoc explainability provides insights into the decision-making process after the trained ML model has made predictions.
Causal ML, on the other hand, focuses on understanding cause-and-effect relationships generating explanations earlier in the AI pipeline, allowing them to impose rules and restrictions during the model building.
With Artificial Intelligence (AI) transforming almost every domain of applications related to automated decision-making processes, it is crucial to design models that are transparent and interpretable. Fields like Causal Machine Learning (Causal ML) and Explainable AI (XAI) provide ways to improve the performance of AI models beyond finding patterns and correlations in data, by understanding how AI reasons, learns and makes decisions.
XAI employs post-hoc explainability techniques generating model-agnostic explanations for feature importance and interaction effects. The disadvantage here is that post-hoc explainability provides insights into the decision-making process after the trained ML model has made predictions.
Causal ML, on the other hand, focuses on understanding cause-and-effect relationships generating explanations earlier in the AI pipeline, allowing them to impose rules and restrictions during the model building.
The advantages of Causal ML or Causal AI are obvious:
Recommends specific actions to change outcomes, going beyond prediction.
Enables feasible bias detection
Offers robustness to changes due to its understanding of cause-and-effect
As an example of advanced performance, the Insurance sector may highly benefit from the Causal AI ability to understand why things happen: no more predicting the customer behavior but understand the reasons behind it. Methods for detecting fraud and managing risk can be effectively designed, while the analysis of causal relationships in claim histories and risk factors is able to reveal feature contributions and boundaries that highlight the fraudulent indicators.
The numbers show a growing influence of AI in the insurance industry: valued at 8.13$ billion in 2024 and (projected by Precedence Research) at 141.44$ billion in 2034. Operations like faster claims processing, error-free results, personalized insurance products by analyzing customer data are some of the benefits that Causal AI can provide. The foresight may go beyond prediction ensuring high performance to strategic planning of insurance companies that will adopt this kind of intelligence.
The advantages of Causal ML or Causal AI are obvious:
Recommends specific actions to change outcomes, going beyond prediction.
Enables feasible bias detection
Offers robustness to changes due to its understanding of cause-and-effect
As an example of advanced performance, the Insurance sector may highly benefit from the Causal AI ability to understand why things happen: no more predicting the customer behavior but understand the reasons behind it. Methods for detecting fraud and managing risk can be effectively designed, while the analysis of causal relationships in claim histories and risk factors is able to reveal feature contributions and boundaries that highlight the fraudulent indicators.
The numbers show a growing influence of AI in the insurance industry: valued at 8.13$ billion in 2024 and (projected by Precedence Research) at 141.44$ billion in 2034. Operations like faster claims processing, error-free results, personalized insurance products by analyzing customer data are some of the benefits that Causal AI can provide. The foresight may go beyond prediction ensuring high performance to strategic planning of insurance companies that will adopt this kind of intelligence.
The advantages of Causal ML or Causal AI are obvious:
Recommends specific actions to change outcomes, going beyond prediction.
Enables feasible bias detection
Offers robustness to changes due to its understanding of cause-and-effect
As an example of advanced performance, the Insurance sector may highly benefit from the Causal AI ability to understand why things happen: no more predicting the customer behavior but understand the reasons behind it. Methods for detecting fraud and managing risk can be effectively designed, while the analysis of causal relationships in claim histories and risk factors is able to reveal feature contributions and boundaries that highlight the fraudulent indicators.
The numbers show a growing influence of AI in the insurance industry: valued at 8.13$ billion in 2024 and (projected by Precedence Research) at 141.44$ billion in 2034. Operations like faster claims processing, error-free results, personalized insurance products by analyzing customer data are some of the benefits that Causal AI can provide. The foresight may go beyond prediction ensuring high performance to strategic planning of insurance companies that will adopt this kind of intelligence.



Expanding our view of the business ecosystem, it becomes evident that leveraging causal insights for business risk management can significantly enhance profitability. For instance, in anomaly detection, Causal AI can pinpoint unusual patterns indicative of fraud or risk, thereby highlighting vulnerable business sectors. Furthermore, by uncovering the root causes of risks, businesses gain the crucial insights needed to develop effective mitigation strategies and accurately predict the impact of various interventions. In business analytics, the deep understanding gained from smaller, specific datasets through causal analysis can boost AI model accuracy, potentially lessening the dependence on big data.
Naturally, there are challenges in the implementation of Causal AI models that have to be taken into consideration. Sometimes the causality may be too complex to transfer into deep learning architectures. Any initial assumptions about the general causality of the use case must be highly accurate because, if not, they may lead to misleading insights and so to failed decisions. Also, the required knowledge for the development of Causal AI models is both technical and domain-specific and more demanding than building traditional correlation-based models.
Since the ability to accurately identify causality will become increasingly important, building trust in AI models is paramount. Causal approaches that enable better decision-making, explaining what, why and how outcomes happen, will be able to drive solutions and strategies that will optimize performance and reduce business risk.
Expanding our view of the business ecosystem, it becomes evident that leveraging causal insights for business risk management can significantly enhance profitability. For instance, in anomaly detection, Causal AI can pinpoint unusual patterns indicative of fraud or risk, thereby highlighting vulnerable business sectors. Furthermore, by uncovering the root causes of risks, businesses gain the crucial insights needed to develop effective mitigation strategies and accurately predict the impact of various interventions. In business analytics, the deep understanding gained from smaller, specific datasets through causal analysis can boost AI model accuracy, potentially lessening the dependence on big data.
Naturally, there are challenges in the implementation of Causal AI models that have to be taken into consideration. Sometimes the causality may be too complex to transfer into deep learning architectures. Any initial assumptions about the general causality of the use case must be highly accurate because, if not, they may lead to misleading insights and so to failed decisions. Also, the required knowledge for the development of Causal AI models is both technical and domain-specific and more demanding than building traditional correlation-based models.
Since the ability to accurately identify causality will become increasingly important, building trust in AI models is paramount. Causal approaches that enable better decision-making, explaining what, why and how outcomes happen, will be able to drive solutions and strategies that will optimize performance and reduce business risk.
Expanding our view of the business ecosystem, it becomes evident that leveraging causal insights for business risk management can significantly enhance profitability. For instance, in anomaly detection, Causal AI can pinpoint unusual patterns indicative of fraud or risk, thereby highlighting vulnerable business sectors. Furthermore, by uncovering the root causes of risks, businesses gain the crucial insights needed to develop effective mitigation strategies and accurately predict the impact of various interventions. In business analytics, the deep understanding gained from smaller, specific datasets through causal analysis can boost AI model accuracy, potentially lessening the dependence on big data.
Naturally, there are challenges in the implementation of Causal AI models that have to be taken into consideration. Sometimes the causality may be too complex to transfer into deep learning architectures. Any initial assumptions about the general causality of the use case must be highly accurate because, if not, they may lead to misleading insights and so to failed decisions. Also, the required knowledge for the development of Causal AI models is both technical and domain-specific and more demanding than building traditional correlation-based models.
Since the ability to accurately identify causality will become increasingly important, building trust in AI models is paramount. Causal approaches that enable better decision-making, explaining what, why and how outcomes happen, will be able to drive solutions and strategies that will optimize performance and reduce business risk.
Διαβάστε επίσης...
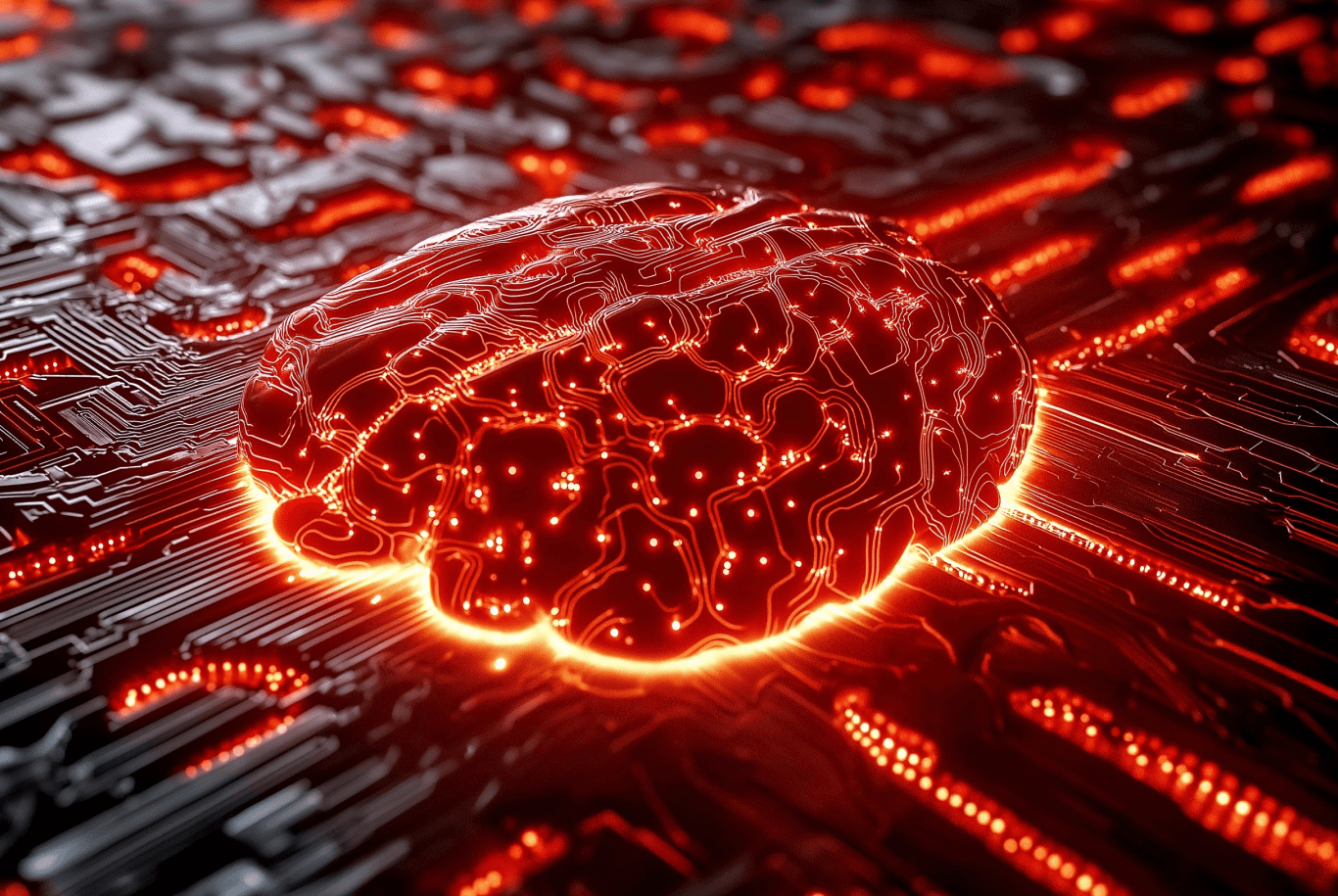
21 Νοε 2024
EU AI ACT: A comprehensive overview of the new AI regulation framework
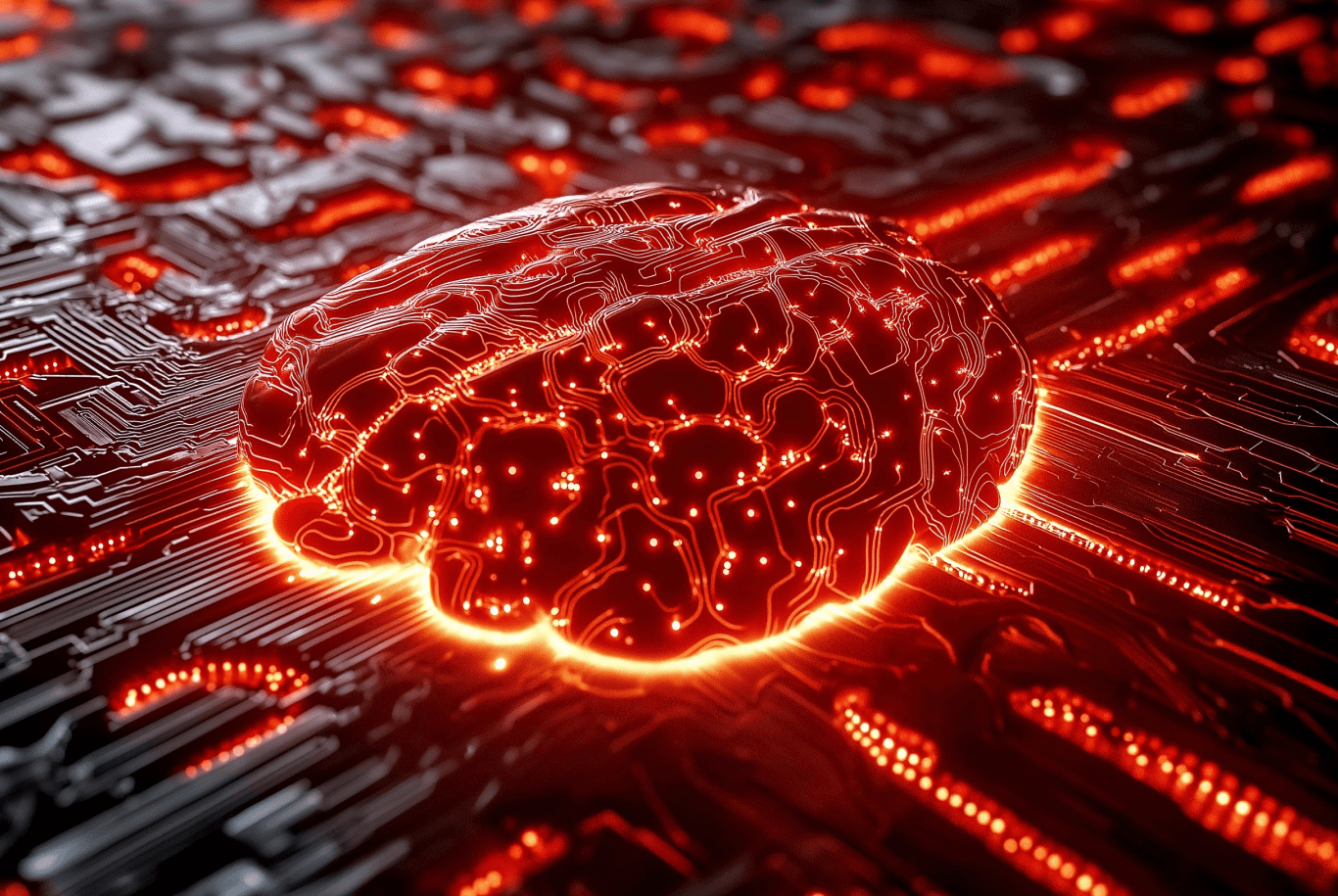
21 Νοε 2024
EU AI ACT: A comprehensive overview of the new AI regulation framework
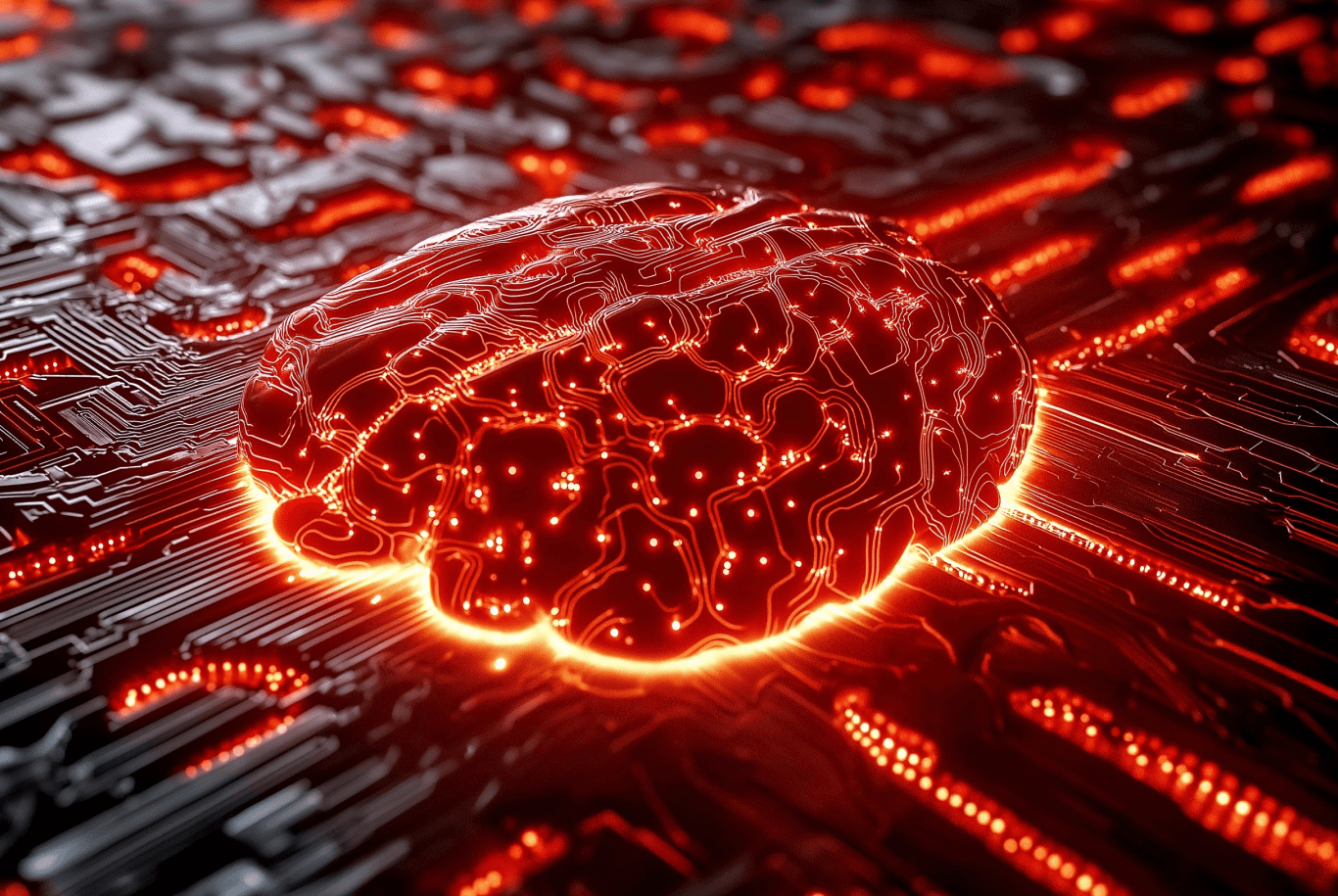
21 Νοε 2024
EU AI ACT: A comprehensive overview of the new AI regulation framework
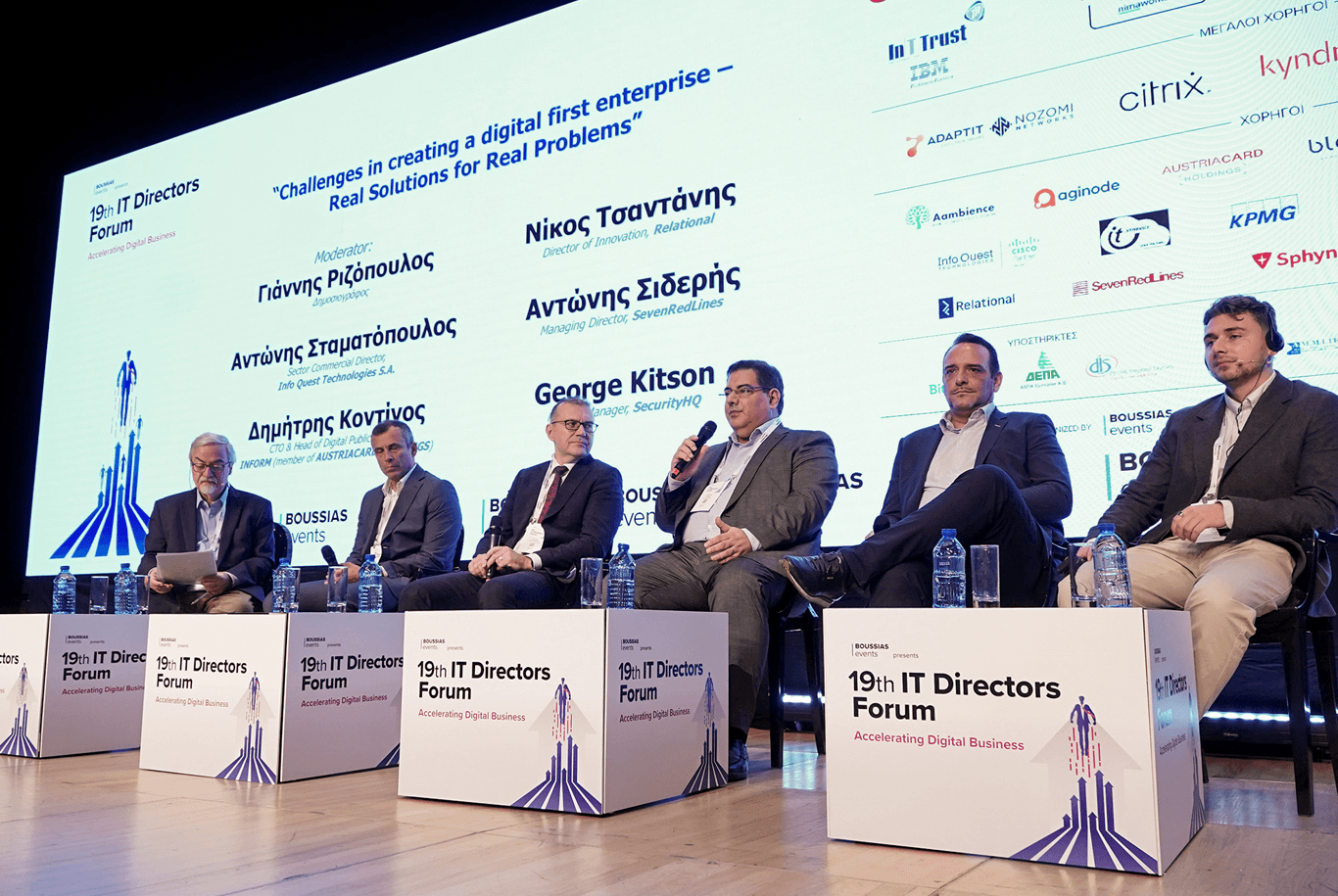
16 Οκτ 2024
Η Seven Red Lines χορηγός στο 19ο IT Directors Forum
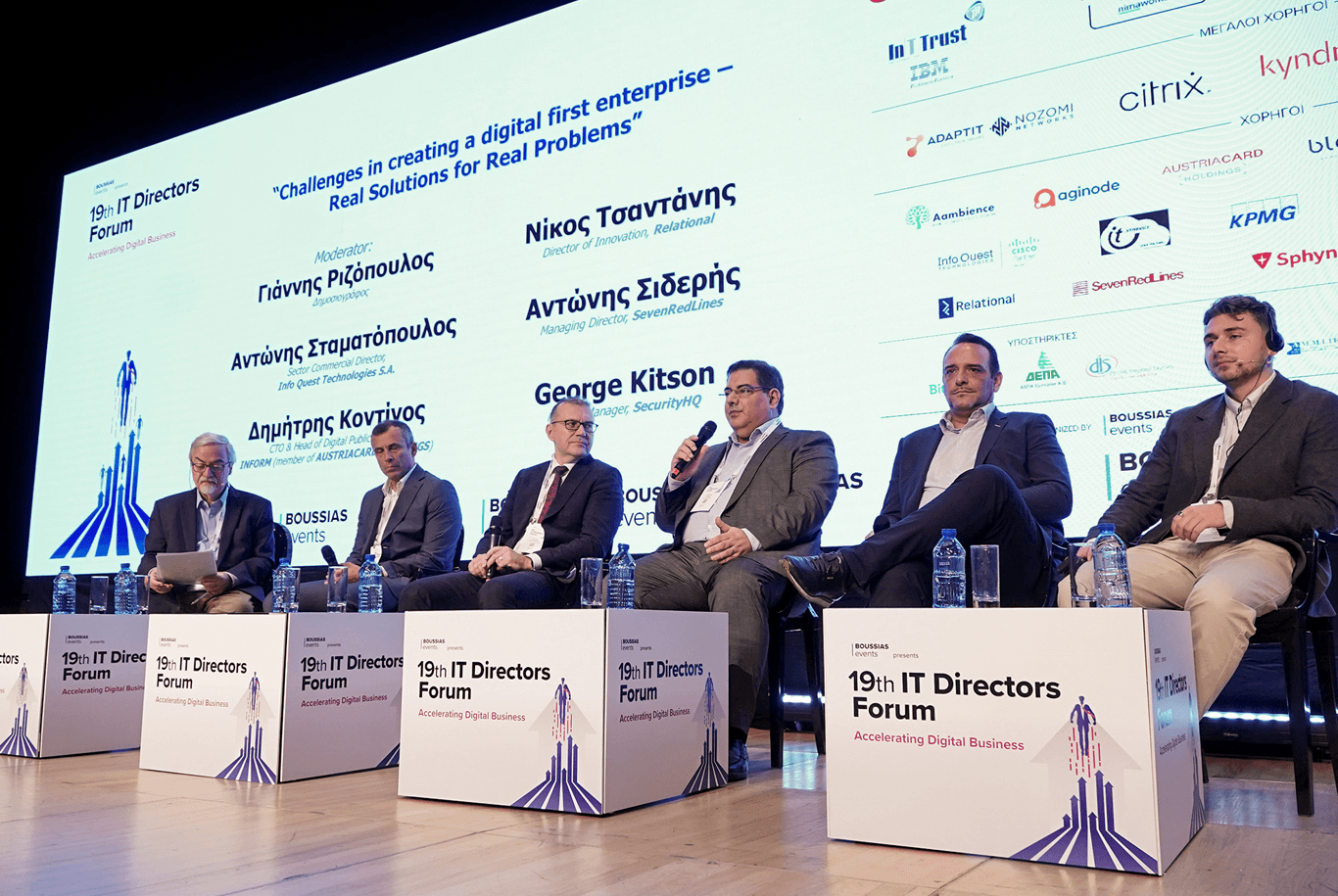
16 Οκτ 2024
Η Seven Red Lines χορηγός στο 19ο IT Directors Forum
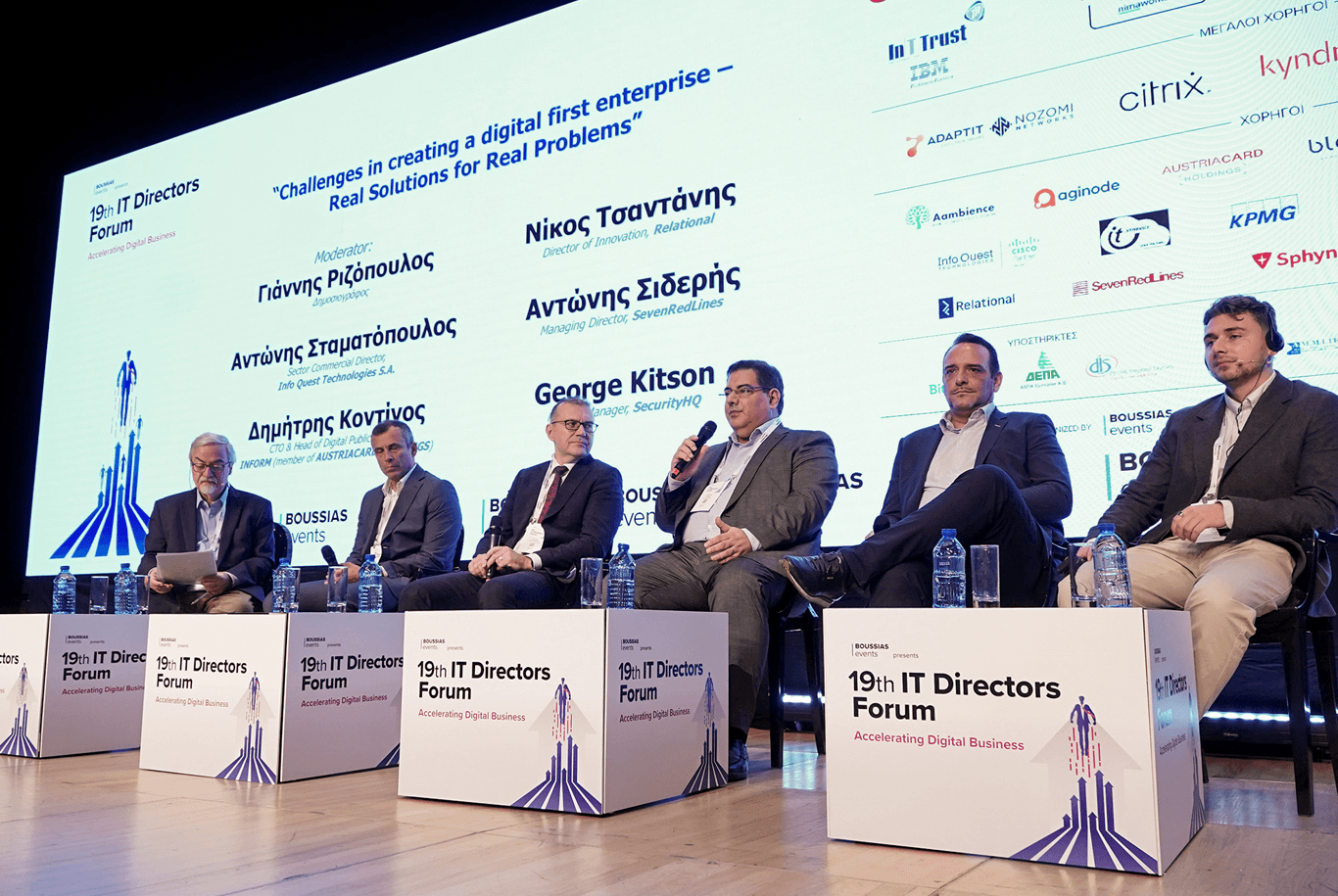
16 Οκτ 2024
Η Seven Red Lines χορηγός στο 19ο IT Directors Forum
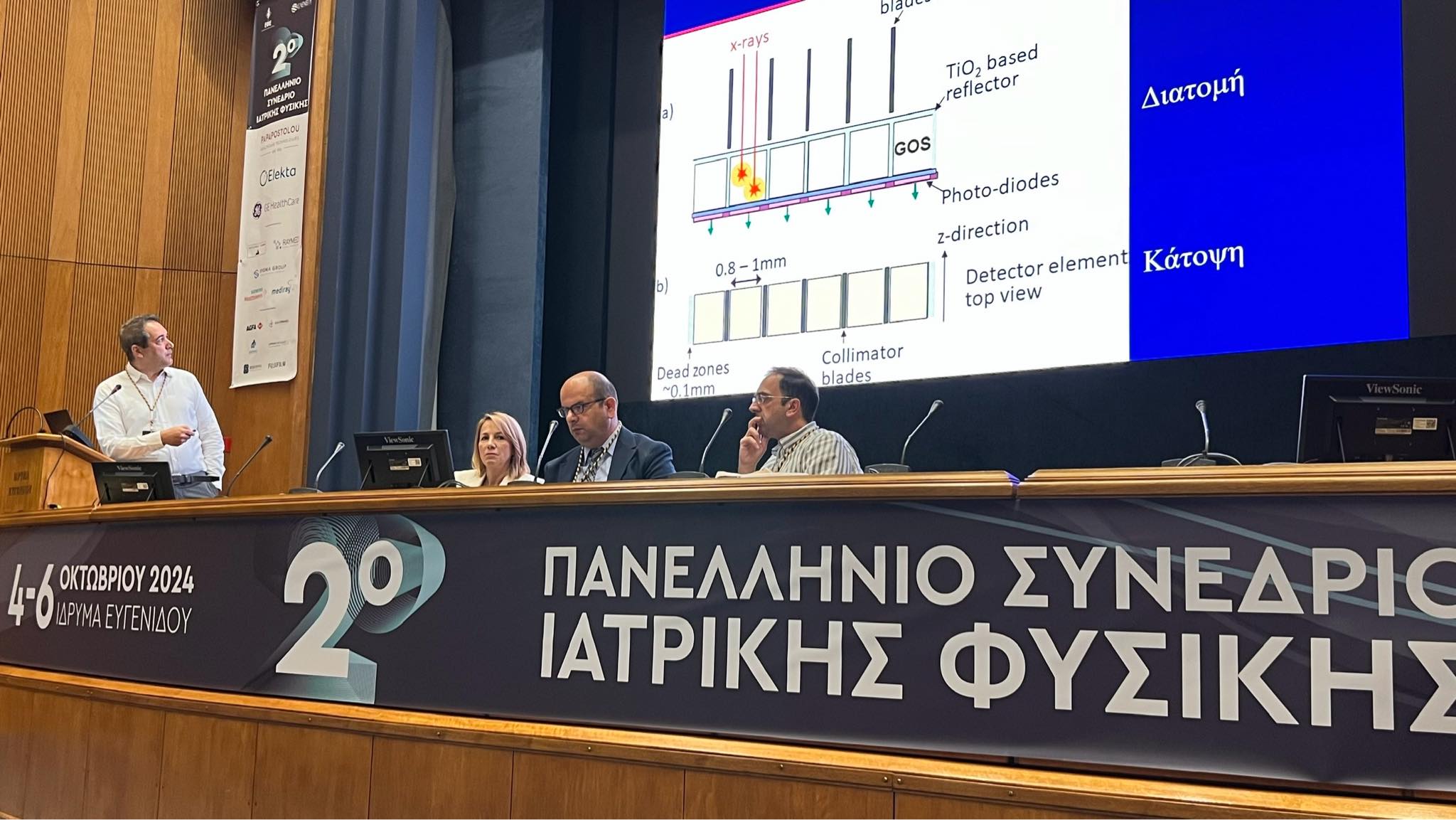
9 Οκτ 2024
Ανάλυση ιατρικών δεδομένων: Παρουσίαση εργασίας στο 2ο Πανελλήνιο Συνέδριο Ιατρικής Φυσικής
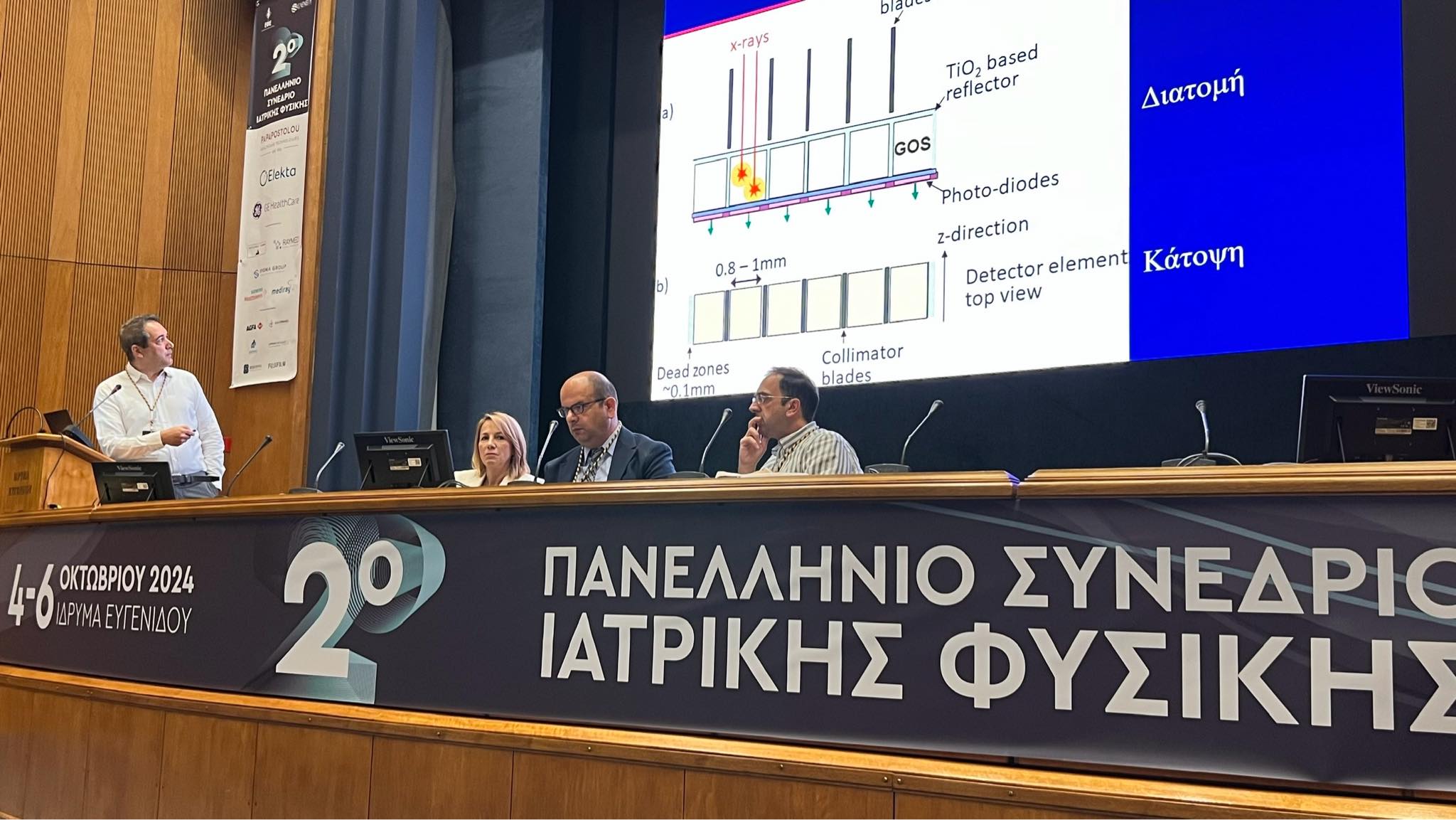
9 Οκτ 2024
Ανάλυση ιατρικών δεδομένων: Παρουσίαση εργασίας στο 2ο Πανελλήνιο Συνέδριο Ιατρικής Φυσικής
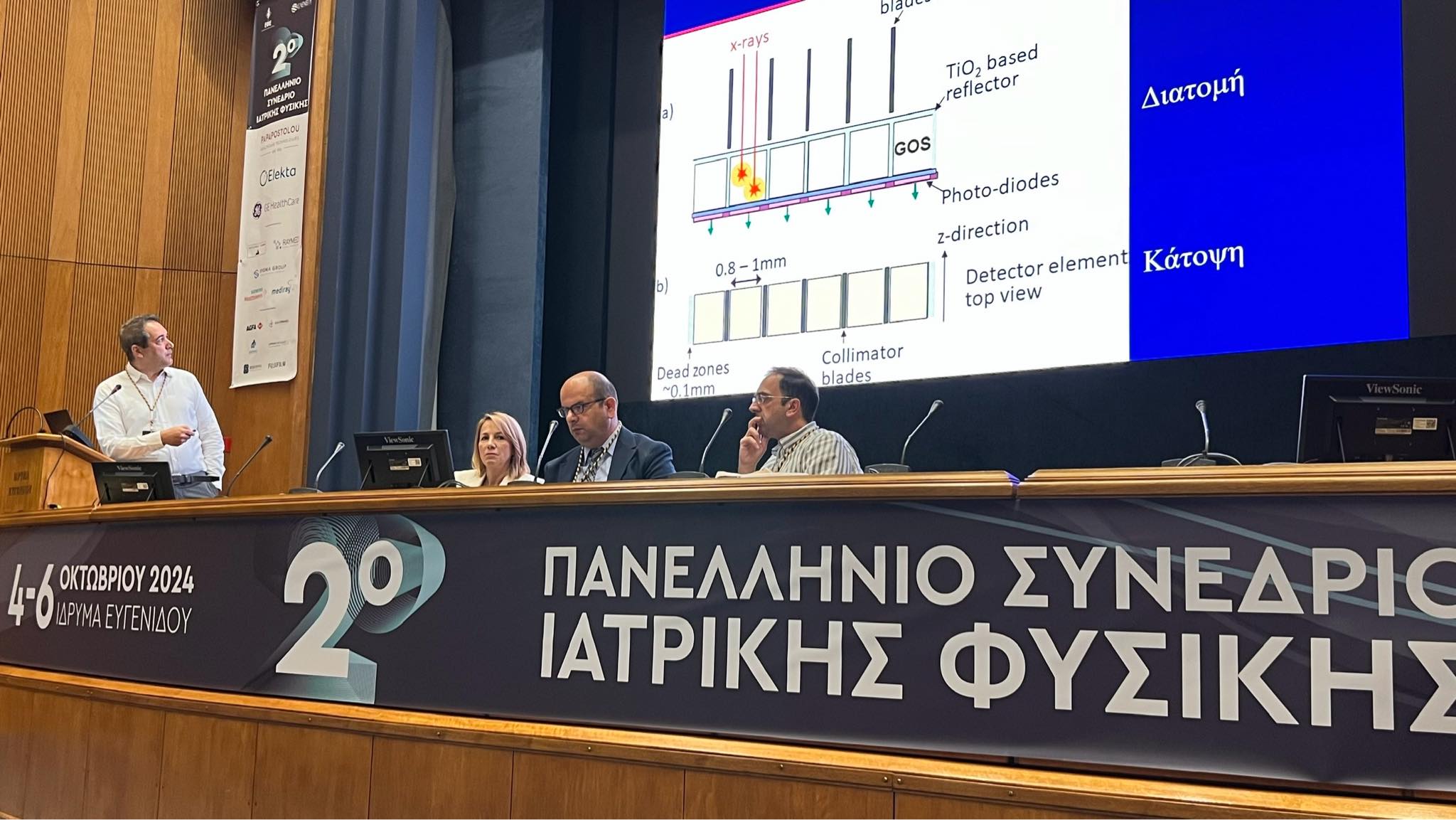
9 Οκτ 2024